Materialize: Querying JSON files
I recently learnt about Materialize, a SQL streaming database, via their Series B fundraising announcement, and thought I’d take it for a spin.
My go-to dataset for new databases is Strava, an app that I use to record my runs. It has an API that returns a JSON representation of each run, containing information like the distance covered, elapsed time, heart rate metrics, and more.
I’ve extracted my latest 30 activities to a file in the JSON lines format and in this post we’re going to analyse that data using Materialize.
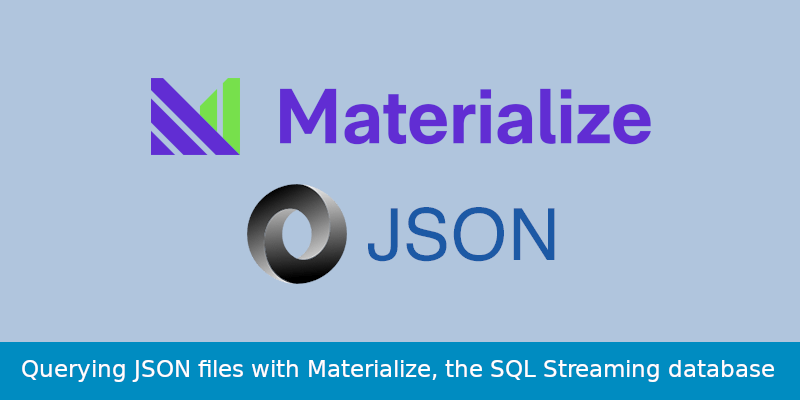
The data is stored in the activities-clean.json
file on the mneedham/materialize-sandbox repository.
Below is an example of the first line in this file:
{"resource_state":2,"athlete":{"id":6958432,"resource_state":1},"name":"Morning Run","distance":14175.1,"moving_time":4222,"elapsed_time":4391,"total_elevation_gain":105.4,"type":"Run","workout_type":null,"id":4470124807,"upload_id":4776694342,"start_date":"2020-12-14T05:31:43Z","start_date_local":"2020-12-14T05:31:43Z","timezone":"(GMT+00:00) Europe/London","utc_offset":0,"location_city":null,"location_state":null,"location_country":"United Kingdom","achievement_count":0,"kudos_count":2,"comment_count":0,"athlete_count":1,"photo_count":0,"trainer":false,"commute":false,"manual":false,"private":false,"visibility":"everyone","flagged":false,"gear_id":"g7064038","from_accepted_tag":false,"upload_id_str":"4776694342","average_speed":3.357,"max_speed":4.3,"average_cadence":87.4,"has_heartrate":true,"average_heartrate":150.3,"max_heartrate":168,"heartrate_opt_out":false,"display_hide_heartrate_option":true,"elev_high":76.2,"elev_low":25,"pr_count":0,"total_photo_count":0,"has_kudoed":false,"suffer_score":116}
Connecting to Materialize
We’re going to query that data using Materialize, which we’ll set up using the following Docker Compose configuration:
version: '3'
services:
materialize:
image: materialize/materialized:v0.5.3
container_name: "materialize-sandbox-docker-compose"
volumes:
- ./data:/data
ports:
- "6875:6875"
The data
directory containing the activities-clean.json
file is in the mneedham/materialize-sandbox/strava GitHub repository.
The repository also contains setup instructions.
Once we’ve cloned that repository, we can launch Materialize by running the following command:
docker-compose up
We should see the following output:
Dec 16 16:13:15.027 INFO materialized: booting server
materialized v0.5.3 (f56c5a8a3)
OpenSSL 1.1.1g 21 Apr 2020
librdkafka v1.5.0
....
=======================================================================
Thank you for trying Materialize!
We are interested in any and all feedback you have, which may be able
to improve both our software and your queries! Please reach out at:
Web: https://materialize.com
GitHub issues: https://github.com/MaterializeInc/materialize/issues
Email: support@materialize.io
Twitter: @MaterializeInc
=======================================================================
materialized v0.5.3 (f56c5a8a3) listening on 0.0.0.0:6875...
We’re now ready to connect to Materialize, which we can do using the psql
CLI tool:
psql -h localhost -p 6875 materialize
psql (12.5 (Ubuntu 12.5-0ubuntu0.20.04.1), server 9.5.0)
Type "help" for help.
materialize=>
Creating a materialized view
To query this file using Materialize we’ll need to create a source around the file and then a materialized view on top of that source. My understanding of the architecture at a high level is shown in the diagram below:

Let’s now create a source on top of the file.
We can do this using the CREATE SOURCE
clause, as shown below:
CREATE SOURCE activities
FROM FILE '/data/activities-clean.json' FORMAT TEXT;
We need to indicate where the file lives on the server, as well as the format of its contents.
We also need to specify the format of the file, which in this case is TEXT
.
We’ll be able to describe the JSON structure in more detail when we create the view.
We can describe the source by running the following query:
SHOW COLUMNS FROM activities;
name | nullable | type |
---|---|---|
mz_line_no |
f |
int8 |
text |
f |
text |
So the main field we’re interested in is the text
one, which contains our JSON documents.
Next we’re going to create a materialized view, in which we can use JSON functions to extract fields from the JSON documents and cast them to appropriate types.
By default fields are imported as the text
type.
We can pull out the interesting data from the activities by creating the following materialized view:
CREATE MATERIALIZED VIEW activities_view AS
SELECT (val->>'id')::float::bigint AS id,
(val->>'distance')::float AS distance,
(val->>'moving_time')::float::int AS moving_time,
(val->>'elapsed_time')::float::int AS elapsed_time,
(val->>'total_elevation_gain')::float AS total_elevation_gain,
(val->>'elev_high')::float AS elev_high,
(val->>'elev_low')::float AS elev_low,
(val->>'average_speed')::float AS average_speed,
(val->>'max_speed')::float AS max_speed,
(val->>'average_heartrate')::float AS average_heartrate,
(val->>'max_heartrate')::float AS max_heartrate,
(val->>'start_date')::timestamp AS start_date
FROM (SELECT text::json AS val FROM activities);
While I was working out which fields to extract, I found myself repeatedly creating and dropping the view.
We can drop the view using the DROP VIEW
clause:
DROP VIEW activities_view;
And once we’re happy with it, we can use the SHOW COLUMNS
clause to describe the view, as shown below:
SHOW COLUMNS FROM activities_view;
name | nullable | type |
---|---|---|
average_heartrate |
t |
float8 |
average_speed |
t |
float8 |
distance |
t |
float8 |
elapsed_time |
t |
int4 |
elev_high |
t |
float8 |
elev_low |
t |
float8 |
id |
t |
int8 |
max_heartrate |
t |
float8 |
max_speed |
t |
float8 |
moving_time |
t |
int4 |
start_date |
t |
timestamp |
total_elevation_gain |
t |
float8 |
Querying a materialized view
Ok, now it’s time for some fun, let’s query the materialized view that we’ve just created.
We’ll start with a SQL query that returns the latest 10 activities, sorted in descending order by start_date
:
SELECT *
FROM activities_view
ORDER BY start_date DESC
LIMIT 10;
id | distance | moving_time | elapsed_time | total_elevation_gain | elev_high | elev_low | average_speed | max_speed | average_heartrate | max_heartrate | start_date |
---|---|---|---|---|---|---|---|---|---|---|---|
470124807 |
14175.1 |
4222 |
4391 |
105.4 |
76.2 |
25 |
3.357 |
4.3 |
150.3 |
168 |
2020-12-14 05:31:43 |
460808499 |
13132.6 |
3794 |
3935 |
81.4 |
67.4 |
25 |
3.461 |
4.6 |
147.3 |
163 |
2020-12-12 06:28:06 |
456998655 |
12244.8 |
3580 |
3643 |
74.4 |
67.4 |
25 |
3.42 |
4.4 |
156.2 |
190 |
2020-12-11 04:15:32 |
448905703 |
12304.8 |
3567 |
3889 |
87.7 |
69.9 |
25 |
3.45 |
4.4 |
153.5 |
182 |
2020-12-09 05:17:29 |
440554208 |
12239.9 |
3856 |
3987 |
87 |
69.8 |
28.1 |
3.174 |
4 |
154.4 |
178 |
2020-12-07 05:38:44 |
431429475 |
13116.8 |
3992 |
4049 |
111.8 |
122.3 |
57 |
3.286 |
4.3 |
156.1 |
185 |
2020-12-05 08:41:17 |
427493338 |
12253.2 |
3756 |
3814 |
73.3 |
67.4 |
29.1 |
3.262 |
4.5 |
158.8 |
182 |
2020-12-04 05:33:21 |
419097799 |
12305 |
3852 |
4013 |
93.9 |
74.6 |
29.6 |
3.194 |
4.6 |
143.5 |
174 |
2020-12-02 05:36:33 |
410708776 |
10916.3 |
3456 |
3645 |
87.8 |
67.3 |
22.9 |
3.159 |
4.1 |
145.7 |
178 |
2020-11-30 05:40:06 |
400404590 |
12926.9 |
4142 |
4243 |
123.1 |
74.6 |
28.5 |
3.121 |
6.4 |
150.6 |
193 |
2020-11-28 07:17:00 |
We can use all the SQL aggregation functions that we’re used to.
So if we wanted to work out the distance run, grouped by month, we could write the following query that uses the date_trunc
function:
SELECT date_trunc('month', start_date) AS month,
sum(distance) AS totalDistance
FROM activities_view
GROUP BY month
ORDER BY month;
month | totaldistance |
---|---|
2020-10-01 00:00:00 |
75149.69999992847 |
2020-11-01 00:00:00 |
194009.49999952316 |
2020-12-01 00:00:00 |
101772.19999974966 |
I ran a lot more in November than the other two months, but admittedly we don’t have complete data for October or December (so far). If we pull in all that data, the total distance covered would likely be similar.
What about the fastest run and the pace per mile on that run?
We can compute with a little help from the to_char
function:
SELECT start_date, distance,
to_char(to_timestamp(moving_time / distance * 1609.34), 'MI:SS') AS pacePerMile,
to_char(to_timestamp(moving_time), 'HH24:MI:SS') AS time
FROM activities_view
ORDER BY moving_time / distance
LIMIT 10;
start_date | distance | pacepermile | time |
---|---|---|---|
2020-12-12 06:28:06 |
13132.6 |
07:44 |
01:03:14 |
2020-12-09 05:17:29 |
12304.8 |
07:46 |
00:59:27 |
2020-10-21 04:34:38 |
12624.5 |
07:48 |
01:01:14 |
2020-10-23 03:49:00 |
13762.7 |
07:49 |
01:06:57 |
2020-12-11 04:15:32 |
12244.8 |
07:50 |
00:59:40 |
2020-12-14 05:31:43 |
14175.1 |
07:59 |
01:10:22 |
2020-10-24 06:04:41 |
13703.4 |
08:01 |
01:08:21 |
2020-10-30 05:43:05 |
8172.7 |
08:02 |
00:40:49 |
2020-10-28 05:33:27 |
14494.7 |
08:05 |
01:12:52 |
2020-11-18 05:42:50 |
11968.1 |
08:06 |
01:00:16 |
It looks like my fastest run was just a few days ago, but I have had a few others that were at a similar pace.
We could combine the previous two queries together to find the fastest pace per month, as shown in the following query:
SELECT to_char(date_trunc('month', start_date), 'YYYY-MM') AS month,
to_char(to_timestamp(min(moving_time / distance * 1609.34)), 'MI:SS') AS pacePerMile,
to_char(to_timestamp(min(moving_time)), 'HH24:MI:SS') AS time
FROM activities_view
GROUP BY month;
month | pacepermile | time |
---|---|---|
2020-10 |
07:48 |
00:40:49 |
2020-11 |
08:06 |
00:39:39 |
2020-12 |
07:44 |
00:59:27 |
If we wanted to also show the average pace per mile, we could do this using the avg
function instead of the min
function on the 2nd line of the query:
SELECT to_char(date_trunc('month', start_date), 'YYYY-MM') AS month,
to_char(to_timestamp(min(moving_time / distance * 1609.34)), 'MI:SS') AS bestPacePerMile,
to_char(to_timestamp(avg(moving_time / distance * 1609.34)), 'MI:SS') AS averagePacePerMile
FROM activities_view
GROUP BY month;
month | bestpacepermile | averagepacepermile |
---|---|---|
2020-10 |
07:48 |
08:00 |
2020-11 |
08:06 |
08:23 |
2020-12 |
07:44 |
08:04 |
In summary
I’ve only skimmed the surface of what we can do with Materialize. From my understanding, a more common use case is to execute SQL queries over streaming data, whereas what we have here is a flat file with static content. I guess querying streaming data is the next thing for me to explore!
About the author
I'm currently working on short form content at ClickHouse. I publish short 5 minute videos showing how to solve data problems on YouTube @LearnDataWithMark. I previously worked on graph analytics at Neo4j, where I also co-authored the O'Reilly Graph Algorithms Book with Amy Hodler.